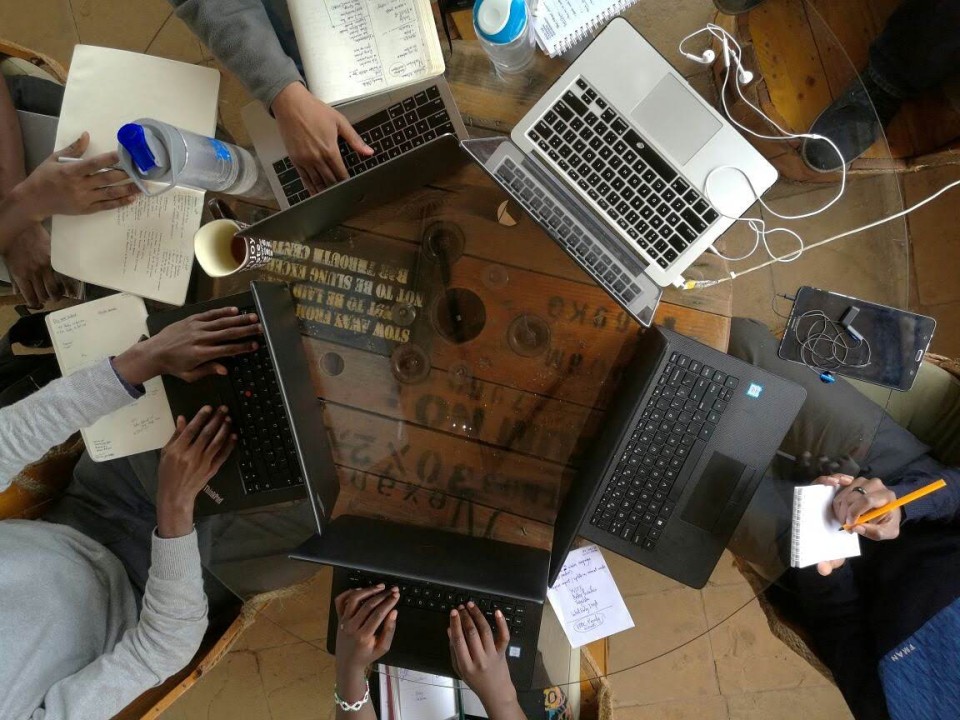
Understanding Data Science As a Service
Big data is here to stay. Organizations have more data than ever before, and in a world overloaded with information, it’s hard to imagine working with a data set that isn’t “big” anymore.
In general, this is good news. But large data sets can be tricky to process correctly without the right tools and resources. Many companies are facing serious challenges in finding ways to process these volumes of data to glean actionable, strategic insights. In fact, more than 50 percent of enterprises surveyed in a 2013 Gartner report stated they didn’t know how to get value out of big data.
For many, the intuitive solution to this problem is to hire a data scientist to parse this data. But this solution has some significant barriers to entry for many organizations. For one, data scientists are expensive. They have a very specific and in-demand skillset, for which they are compensated appropriately. And although they add value to a team, not all businesses have the resources to hire someone dedicated specifically to data science. Even if the resources did exist, the high demand currently being placed on this position means that many organizations are hard-pressed to find a data scientist to hire. The fact is, there is a dearth of qualified people to feed the growing demand for data scientist roles.
But another solution for organizations processing big data for strategic insights is even simpler than hiring a data scientist and can be done by surfacing analytics in workflows that employees are already familiar with in order to make those workflows richer and more relevant. The simplest way of doing this, rather than requiring that teams hone new skillsets, is data science a service.
What is Data Science as a Service?
The notion of data science as a service is in its infancy but starting to gain momentum. Internet searches reveal several companies selling “data science as a service,” but offer no definition. Many of these companies promise to help handle big data without saying how. This certainly makes data science as a service seem like an unknown quantity.
However, data science as a service isn’t so mysterious: it can be defined as user-friendly analytics programs that surface analytics data to business users within their current workflows. It’s often implemented using external tools and software that can automate the analytics process by integrating with an organization’s data storage and business solutions to quickly analyze, shape, and disseminate information throughout the organization.
Some enterprises are already doing this today. Data science as a service tools have been developed with a non-technical worker in mind. By focusing on users’ business needs rather than complex statistical models, these tools return information that can be used in real time.
Here’s an example: For a call center employee to give the best service to a customer who has called in, it would be very helpful if, simply by entering some basic information – name, email, or phone number, the representative could see the customer’s recent purchase history. But what if he could see more than that? What if the rep could see how the customer typically rates the company in surveys? The customer’s return history? The average dollar amount the customer spends with the company or the frequency with which she makes purchases?
This type of information could quickly give the call center rep insight into not only what the customer’s issue might be, but also the customer’s frame of mind when calling. This example of surfacing analytics into existing workflows is just one of the ways that data science as a service helps make analytics more accessible to employees at all levels of the enterprise.
Some organizations may already be doing this to some degree with in-house tools. But compared to data science as a service, these in-house tools fall short in that they are not able to make information available nearly as flexibly or quickly. Data science as a service tools have the ability to quickly shape the information within the service as the process or workflow changes, as quickly as seconds after that data is collected. Some systems even have the ability to respond to questions about insights in milliseconds, ensuring that insights can be acted upon instantly within external workflows. The result is that the call center employee can depend on immediately actionable and up-to-date information that’s specifically tailored to the task at hand. The key to successfully democratizing data science insights is having the ability to constantly shape and refresh the data-driven view so access to the information happens in real-time, precisely when it’s needed.
By introducing data science as a service tools into employees’ everyday workflows that give them insights into what they are doing and how they can improve things, companies can make their employees much more strategic and effective in their work.
Transform Your Team with Data Science as a Service
Hiring someone dedicated specifically to the task of data science and parsing data is no longer enough to share those insights at scale. Data science can work as a service throughout an organization by integrating with existing workflows, and it’s important to understand how this works in meeting the needs of the business.
At a high level, CMOs need what data science provides – a better understanding of customers – and they are relying on technology to get there. In fact, a recent Gartner report predicts that CMOs soon will spend more on technology than the CIO. And it’s not just the CMO who needs data insights – so do thousands of others within the organization.
New tools that make data science accessible to people at all levels of the organization provide user-friendly interfaces, queries that can be saved to use again and again, easy-to-understand dashboards, and more. These functions allow internal users to gain insights from the company’s data quickly by running queries without needing to know how to run the statistics themselves.
For business analytics to be most effective, it must be democratized. Insights can’t stop at the C-suite. The entire internal team must have access to metrics and analytics to serve clients. And it needs access to this data in real-time. For members of the organization on the front lines of customer interface, a once-a-month report that is funneled from a data scientist to the CEO to the rest of the organization is not enough to enable them to provide the best service to customers and stakeholders. Internal metrics, both on a broad scale and on a client-by-client basis, must be accessible to internal teams at the drop of a hat.
For this to happen, the data science experience must be approachable for less sophisticated analysts within the organization. Web analysts, business analysts – the crowd that helps drive insight for the organization but doesn’t have the education and skills currently demanded by today’s data science toolkits – all must be able to easily understand analytics that relate to them. This happens by creating very simple and quick access to customer analysis reports to get answers to critical business questions without having to do hardcore analysis. Customer segmentation, for example, must be easy enough for a business user to do in a matter of seconds, rather than going through extensive training on a long IT process.
Also, data insights should be surfaced in workflows that otherwise have nothing to do with analysis or data science. It is no longer practical to hire a data scientist or two who then provides insights only to the CMO. Entire teams of people need access to these insights, often instantly. Surfacing analytics organically into workflows provides front-line workers with access to strategic information in real-time. Imagine the possibilities when analytics are an easy-to-consume medium as part of the work process. Marketers at live events can better understand the needs of the person they’re speaking with through access to their history with the company. Content developers can better understand which images are most likely to help make a sale based on past performance during different times of the year.
Data science as a service is a best practice for helping an organization move to the next level, as the tools have the ability to amalgamate data from multiple places in the organization while also democratizing access to data and its insights. This way, big data does not have to be tackled by any one person and everyone can share in its benefits. There’s no simpler way to help both the organization and its customers.